In today's digital transformation world, data has become essential for modern businesses. From providing valuable customer insights to enabling automation, data is a factor in driving business growth and fostering innovation. However, as organizations rely more on data, they must also confront the ethical considerations of data collection, storage, and usage. Ethical data engineering is no longer a choice; it has become a vital business necessity. This article delves into the essential strategies for maintaining ethical data engineering in the contemporary enterprise.
Understanding Ethical Data Engineering
Understanding ethical data engineering is crucial before delving into strategies. Ethical data engineering involves responsibly collecting, storing, processing, and sharing data while respecting privacy, fairness, transparency, and security. It includes creating data pipelines and architectures that prioritize ethical considerations, such as compliance with regulations, data minimization, and protection against biases.
Core Elements of Ethical Data Engineering
Privacy: Ensuring individuals' data is collected and used with consent and protecting it from unauthorized access.
Fairness: Preventing bias in data collection, processing, and decision-making.
Transparency: Providing clear information on data usage and decision-making processes.
Security: Protecting data from breaches, theft, and misuse.
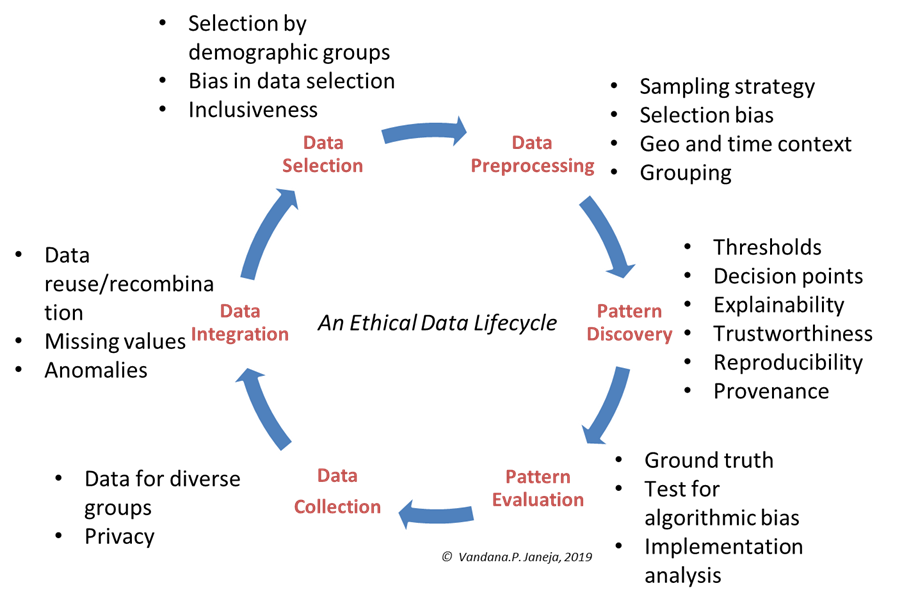
Building a Culture of Data Ethics
A key strategy in ethical data engineering involves establishing a culture of data ethics within the organization. It includes raising awareness and promoting responsibility for ethical data handling across all teams. Leadership requires leading by example and advocating for ethical data practices to set the tone for the organization.
Steps to Foster a Data Ethics Culture
Training and Awareness: Conduct regular training sessions on data ethics, privacy laws, and responsible data usage.
Ethical Guidelines: Develop and disseminate ethical guidelines that outline the principles and practices of data handling.
Data Governance Committees: Establish a dedicated committee to oversee data practices, ensure compliance, and address ethical concerns.
Ensuring Compliance with Data Protection Laws
Compliance with data protection regulations like GDPR, CCPA, and HIPAA is a cornerstone of ethical data engineering. Modern enterprises must understand the legal landscape and implement measures to comply with these laws. It includes obtaining consent for data collection, enabling users to exercise their rights over their data, and maintaining robust data security practices.
Key Compliance Strategies
Data Audits: Regularly conduct data audits to ensure compliance with relevant laws and identify any gaps in data practices.
Consent Management: Implement systems to manage user consent, track preferences, and allow for easy withdrawal of consent.
Data Minimization: Collect only the data necessary for specific purposes and avoid excessive data collection.
Prioritizing Data Privacy and Security
Data privacy and security are integral to ethical data engineering. Protecting user data from breaches and unauthorized access is not just a legal requirement but also a moral obligation. A robust data security strategy involves a combination of technological measures, policy frameworks, and employee training.
Implementing Effective Privacy and Security Measures
Encryption and Anonymization: Use modern encryption techniques to protect data in transit and at rest. Anonymize sensitive data wherever possible to minimize risks.
Access Controls: Implement strict access controls and ensure authorized personnel can access sensitive data.
Regular Security Audits: Conduct security audits and vulnerability assessments to identify and mitigate risks.
Eliminating Bias in Data Processing
Data-driven decision-making is only as good as the data itself. If the data used is biased, the outcomes will also be biased. Ethical data engineering involves identifying and eliminating biases in data collection, processing, and analysis to ensure fairness and impartiality in decision-making.
Strategies to Mitigate Bias
Diverse Data Sources: Ensure data is collected from diverse sources to minimize inherent biases.
Bias Detection Tools: Employ AI and machine learning technologies to identify and rectify prejudices in datasets and algorithms.
Inclusive Data Teams: Encourage diverse teams to work on data projects, as varied perspectives help identify and address potential biases.
Promoting Transparency in Data Practices
Transparency is a critical aspect of ethical data engineering. Users should know how their data is collected, stored, and used. Transparent practices help build trust with customers, stakeholders, and regulatory bodies.
Steps to Enhance Transparency
Data Usage Policies: Communicate data usage policies on websites and applications, explaining what data is collected, why, and how it will be used.
Open Data Practices: Where possible, share non-sensitive data and algorithms with the public to foster innovation and accountability.
Regular Reporting: Provide reports on data practices, including incidents, policy changes, and compliance status.
Embracing Ethical AI and Machine Learning
AI and machine learning (ML) are transforming businesses but come with ethical challenges. From biased algorithms to opaque decision-making processes, there are many risks associated with AI. Ethical data engineering requires the adoption of responsible AI practices.
Practices for Ethical AI
Explainable AI: Use explainable AI models that provide clear, understandable outcomes and decision-making processes.
Algorithmic Accountability: Ensure AI models are tested for fairness and do not discriminate against any group.
Continuous Monitoring: Regularly monitor AI models for biases and unintended consequences, adjusting them as necessary.
Data Governance Frameworks
To manage data ethically, it is crucial to establish a robust data governance framework. This framework must clearly define the roles, responsibilities, and procedures for data management within the organization. It ensures the maintenance of data quality, compliance with regulations, and ethical usage of data.
Elements of a Robust Data Governance Framework
Data Stewardship: Assign data stewards responsible for managing data assets and ensuring they align with ethical guidelines.
Data Quality Standards: Define and enforce data quality standards to ensure accurate and reliable data.
Policy Development: Develop policies that address ethical considerations, such as data retention, deletion, and sharing.
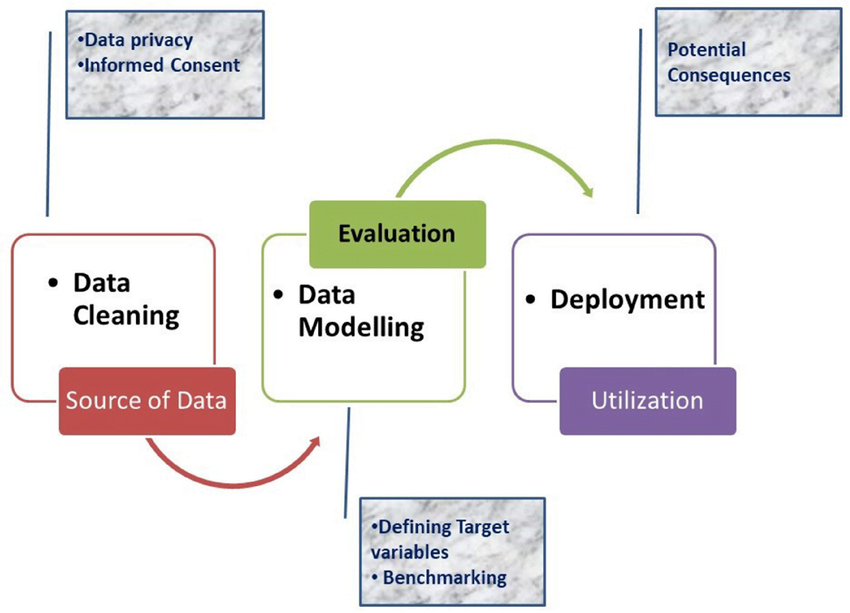
Leveraging Technology for Ethical Data Practices
Ethical data engineering relies heavily on technology. Utilizing different tools and platforms can automate compliance processes, identify biases within data, and bolster data security measures.
Tools and Technologies to Consider
Data Masking Tools: Tools that anonymize or mask sensitive data to protect privacy.
Bias Detection Software: AI-driven tools that analyze datasets and algorithms for biases.
Compliance Management Software: Solutions that automate compliance with data protection laws and regulations.
Measuring the Impact of Ethical Data Practices
Measuring the effectiveness of ethical data engineering strategies is vital for organizations. They should set up key performance indicators (KPIs) to evaluate compliance, data security, bias reduction, and transparency.
Metrics to Track
Data Breach Incidents: Number and severity of data breaches over time.
Compliance Rate: Percentage of data practices that meet regulatory requirements.
Bias Reduction: Improvement in fairness and reduction of biases in data-driven outcomes.
Conclusion: The Future of Ethical Data Engineering
Ethical data engineering involves building a sustainable, responsible approach to data that respects individuals' rights and privacy while driving innovation and growth. As technology evolves, our commitment to ethical data practices must also evolve. Adopting outlined strategies can ensure that data engineering practices are efficient and ethical.
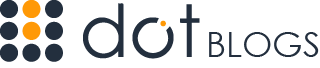
Dot Labs is a leading IT outsourcing firm renowned for its comprehensive services, including cutting-edge software development, meticulous quality assurance, and insightful data analytics. Our team of skilled professionals delivers exceptional nearshoring solutions to companies worldwide, ensuring significant cost savings while maintaining seamless communication and collaboration. Discover the Dot Labs advantage today!
Visit our website: www.dotlabs.ai, for more information on how Dot Labs can help your business with its IT outsourcing needs.
For more informative Blogs on the latest technologies and trends click here