Data engineering is the heart and soul of powering data-driven decisions in today's rapidly digitalized landscape. With the mushrooming of data across industries, traditional workflows in data engineering are frequently overwhelmed with complexity, scalability demands, and time constraints. A groundbreaking technology in reshaping the data engineers design, build, and optimize their workflows is Generative AI.
What is Generative AI?
Generative AI refers to machine learning models, such as GPT (Generative Pre-trained Transformer), trained to generate content—text, code, images, and more. By understanding patterns in large datasets, these models can create high-quality outputs that mimic human creativity and reasoning.
In data engineering, generative AI comes forth as a powerful tool that will automate complex tasks like writing SQL queries, building pipelines, and even recommending optimized architectures.
The Need for Generative AI in Data Engineering
Challenges in Traditional Data Engineering Workflows
Time-Consuming Processes: Writing and debugging ETL scripts, designing data models, and creating dashboards can take weeks or months.
Complexity and Scale: As the size of data grows, traditional workflows are unable to match processing and storage requirements.
Talent Shortage: This kind of data engineer expertise is scarce, leaving a team understaffed and overburdened.
Error-Prone Operations: Manual intervention tends to increase the prospect of error which translates to expensive downstream consequences.
The above issues are addressed by introducing automation, precision, and scalability into workflow with generative AI.
Applications of Generative AI in Data Engineering
Automated Data Pipeline Creation
Generative AI models can comprehend requirements and automatically generate code for building data pipelines. It saves manual coding time and makes sure best practices are implemented.
Example: Engineers use AI-powered tools like dbt (Data Build Tool) with GPT integration to generate SQL queries and data transformations through natural language prompts.
Effective Query Generation
Complex SQL queries are very time-consuming to write manually. Generative AI tools aid in:
Translation of natural language queries into SQL.
Query optimization suggestions.
Debugging of existing queries.
Example: Instead of hand-writing a query, you can ask a generative AI model to "retrieve all customer data from the past year where the purchase value exceeds $500."
Data Quality Assurance
Generative AI helps detect and resolve data inconsistencies, anomalies, and missing values. It may also suggest improvements in the data validation rules.
Impact: Better accuracy and reliability in downstream analytics.
Schema Design and Documentation
Data engineering is invested in the design of schemas. The capabilities of generative AI are:
Recommendation of schema design based on specific use cases.
Automation of documentation process for clear communication and transparency.
Example: GPT-4 can provide schema diagrams or detailed descriptions of tables in a more accessible way to stakeholders.
Increased Collaboration with AI-Driven Insights
Generative AI allows for increased collaboration, summarizing large datasets into insights, generating visualizations, and more.
Example: Looker Studio or Tableau with GPT integrations are examples of AI-powered tools that provide an instant summary of dashboard metrics.
Generative AI Tools in Data Engineering
There are quite a few tools and platforms that use generative AI in data engineering. Here are some of the leading ones:
OpenAI Codex
Generates code snippets for ETL processes.
Integrates seamlessly with IDEs to assist with real-time coding.
DataRobot
DataRobot automates machine learning workflows and helps with data preprocessing and feature engineering.
AI-Powered ETL Tools
Tools such as Matillion and Alteryx incorporate generative AI into ETL workflows to automate the process.
In-house Generative Models
Data engineering can train models specific to organizational needs.
Benefits of Generative AI in Data Engineering
Time-Saving
Automation of repeated tasks frees engineers to do high-value tasks, including architectural design and performance tuning.
Scalability
AI models can process large datasets with ease. This means workflows can be scaled up according to growing business needs.
Accuracy
Generative AI eliminates human errors, providing consistent and reliable results.
Cost Effectiveness
Streamlining workflows saves resources and reduces operational costs for businesses.
Democratization of Data Engineering
Non-technical stakeholders can use natural language queries to interact with data, making data more accessible across teams.
How to Implement Generative AI in Data Engineering
Assess Your Workflow Requirements
Identify areas where automation or optimization would have the most impact, such as ETL processes or data validation.
Select the Appropriate Tools
Identify the suitable AI-enabled platforms for your tech stack and business objectives.
Train Your Team
Upskill your data engineering team to comprehend and apply generative AI effectively.
Pilot, then Scale
Use generative AI for focused use cases to scale up within your organization.
Monitor and Improve
Keep tracking the outputs of the AI and improve its accuracy and performance by modifying models where necessary.
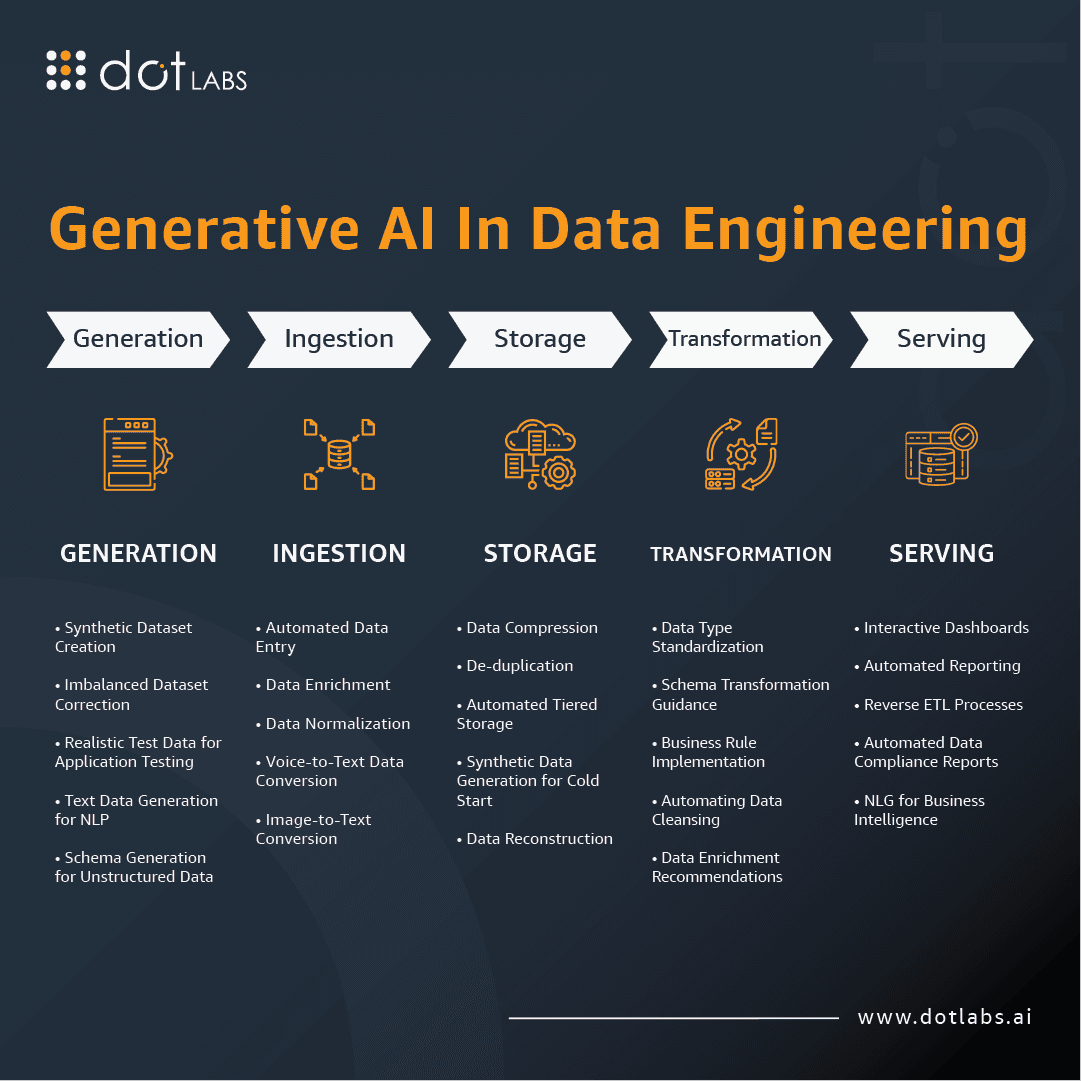
Generative AI in Data Engineering
Trends of Generative AI in the Future of Data Engineering
Several exciting trends are promised by the rapid evolution of generative AI:
Custom AI Models: Organizations will develop bespoke generative models tailored to their unique workflows.
AI-Powered Data Lakes: Generative AI will optimize the creation and management of data lakes, improving data retrieval speed.
Augmented Collaboration: Generative AI will further bridge the gap between technical and non-technical teams, enabling seamless collaboration.
Ethics around AI: Ethical AI will become much more important as AI gets widely adopted prominence of data privacy and mitigation of bias.
Final Thoughts
Generative AI is more than just a tool; it's a revolution in data engineering. It empowers organizations to focus on innovation and strategic growth by automating repetitive tasks, optimizing workflows, and enhancing collaboration. Businesses that aim to stay ahead in this data-driven world can no longer afford not to integrate generative AI into their data engineering workflows.
FAQs
How does generative AI enhance data engineering workflows?
Generative AI can automate coding, query generation, data validation, and schema design to cut down manual efforts and mistakes.
Will generative AI replace data engineers?
No. Generative AI is a productivity-enhancing and efficiency-boosting tool, but human oversight and expertise remain paramount.
What are some tools that can help me incorporate generative AI into my workflows?
OpenAI Codex, DataRobot, and other AI-powered ETL platforms, like Matillion.
Is generative AI safe to use with sensitive data?
Yes, when properly secured, including encryption of data and access controls.
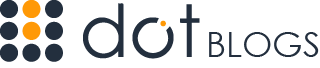
Dot Labs is a leading IT outsourcing firm renowned for its comprehensive services, including cutting-edge software development, meticulous quality assurance, and insightful data analytics. Our team of skilled professionals delivers exceptional nearshoring solutions to companies worldwide, ensuring significant cost savings while maintaining seamless communication and collaboration. Discover the Dot Labs advantage today!
Visit our website: www.dotlabs.ai, for more information on how Dot Labs can help your business with its IT outsourcing needs.
For more informative Blogs on the latest technologies and trends click here