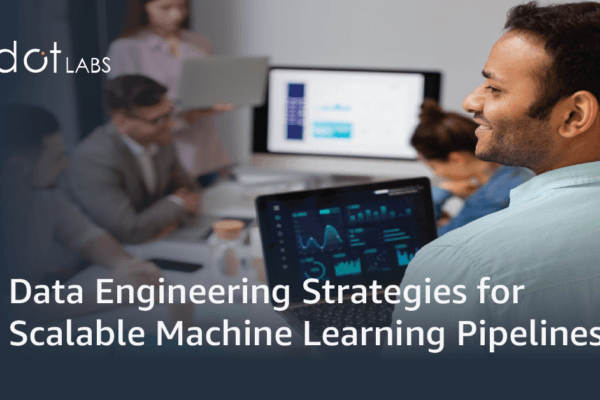
Data Engineering Strategies for Scalable Machine Learning Pipelines
In the era of data-driven innovation, scalable machine learning (ML) pipelines are essential for turning vast datasets into actionable insights. This blog explores key data engineering strategies for building robust ML pipelines, focusing on scalability, data quality, accessibility, and security. Learn how to leverage tools like Apache Spark, Snowflake, and TensorFlow Extended while addressing challenges such as data volume, processing speed, and pipeline maintenance. Embrace best practices and MLOps principles to ensure your AI initiatives remain efficient and impactful as data complexity grows. Optimize your ML pipelines today to stay ahead in the AI revolution.