Artificial Intelligence has gained
significant importance in the past few years. The use of AI has also started in
different engineering fields as well. AI-powered tools can analyze large
volumes of data, identify patterns, and generate optimized ETL workflows
automatically. This automation not only accelerates the data engineering
process but also reduces the risk of human error, thereby increasing overall
data quality and reliability.
Modern data engineering is shifting towards
decentralized and flexible approaches, with the emergence of concepts like Data
Mesh, which advocates for federated data platforms partitioned across the
domains.
The field of data engineering is undergoing profound transformation, thanks to the infusion of Artificial Intelligence (AI). As organizations increasingly recognize the value of data in driving decision-making, AI is emerging as a pivotal tool in optimizing data engineering processes and enhancing data quality.
Automation
of Data Pipelines using AI:
Data engineering often involves the
collection and integration of data from diverse sources, which can be a
labor-intensive and time-consuming task. Traditionally, data engineers had to
manually design and develop complex data pipelines to extract, transform, and
load (ETL) data. However, nowadays Artificial Intelligence (AI), particularly
Machine Learning (ML) algorithms, is being used to automate data extraction,
ingestion, and integration.
Automating data pipelines can be as
straightforward as streamlining moving data from point A to point B or as
complex as aggregating data from multiple sources, transforming it, and storing
it in multiple destinations. With the ability to identify patterns and
relationships in data, AI can streamline these processes, reducing the need for
manual intervention and minimizing errors.
Improved
Data Quality and Cleansing:
High-quality data is the cornerstone of effective data engineering. AI-powered tools are becoming increasingly proficient at data quality assessment and cleansing. These tools can automatically detect and rectify anomalies, outliers, and missing values in datasets, ensuring that the data used for analysis is accurate and reliable. Moreover, AI can enhance data standardization by enforcing consistency in data formats and naming conventions.
Predictive
Analytics for Data Engineering:
AI is not just about automating
tasks; it can also predict data engineering requirements. Machine learning
models can forecast data volume trends, helping organizations scale their
infrastructure proactively. This predictive capability ensures that data engineering
systems can adapt to evolving data needs without delays or capacity issues.
Data
Transformation and Enrichment
Data engineering often involves
complex data transformations and enrichments to make data suitable for
analysis. AI-powered data transformation tools can intelligently process and
structure data, making it easier for data engineers to handle complex data
transformations. Additionally, AI can enrich data by adding valuable context,
such as geospatial information or demographic details, to enhance the depth of
analysis.
Streamlining
ETL Processes
Traditionally, ETL (Extract, Transform, Load) processes have been a core component of data engineering. AI streamlines these processes, making them more efficient and adaptable. AI-driven ETL tools can automatically adjust to changing data sources, making them resilient to alterations in data schema or source structures.
Data
Governance and Compliance
Data governance is a critical aspect of data engineering, especially in industries with rigorous regulations. AI can assist in data lineage tracking, ensuring data is compliant with privacy and security standards. It can also aid in identifying and mitigating potential data risks, helping organizations maintain compliance and minimize data-related vulnerabilities.
Summary:
The AI revolution in data engineering is transforming the way data is collected, integrated, and processed, leading to enhanced data quality, greater efficiency, and improved decision-making. As AI continues to evolve, its impact on data engineering will only become more profound. Organizations that leverage AI in their data engineering processes will be better positioned to unlock the full potential of their data, gain valuable insights, and maintain a competitive edge in the data-driven era. The future of data engineering is undeniably intertwined with the capabilities of Artificial Intelligence, making it an exciting time for the field.
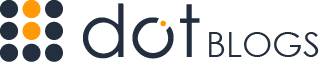
Dot Labs is an IT outsourcing firm that offers a range of services, including software development, quality assurance, and data analytics. With a team of skilled professionals, Dot Labs offers nearshoring services to companies in North America, providing cost savings while ensuring effective communication and collaboration.
Visit our website: www.dotlabs.ai, for more information on how Dot Labs can help your business with its IT outsourcing needs.
For more informative Blogs on the latest technologies and trends click here