What
is data engineering all about?
This is a question that almost everyone has in their mind when they first hear
the term data engineer. To understand the term data engineer or data engineering,
it is better to understand the working of data engineers.
· Extracting and integrating data from a variety of sources such as collecting all
the required data from different resources.
· Preparing
the data for analysis; processing the data by applying suitable transformations
to prepare the data for analysis and other downstream tasks. Includes cleaning,
validating, and transforming data.
· Designing,
building, and maintaining data pipelines that encompass the flow of data from source to
destination.
· Design
and maintain infrastructure for data collection, processing, and storage such as
managing the infrastructure.
Data engineering comprehends the processes
that collect and integrate raw data from various resources into a unified and
accessible data repository, that can be used for analytics and other
applications.
So, we can say that Data Engineering is the discipline that
transforms raw data into a structured, manageable, and actionable format,
making it a critical component in today's data-driven world. It involves
designing and maintaining data pipelines, integrating data from various
sources, and ensuring that the data is in a format that can be easily analyzed
and used by data scientists, analysts, and other stakeholders.
A few years back, the primary focus
was on collecting and assembling the insights from data. However, as the
industry matured, the significance of robust data management and the saying
"Garbage In, Garbage Out" became more obvious.
This shift in perspective has brought the role of data engineers to the forefront, emphasizing the symbiotic relationship between them and data scientists.
The process of data engineering
typically includes the following key steps:
Data
Ingestion:
This stage entails obtaining information from a variety of sources,
including log files, databases, and APIs. Setting up procedures and
systems for dependable data collection and intake is the responsibility of
data engineers.
Data
Transformation:
Analyzing raw data can be difficult since it is frequently disorganized or
unstructured. Data is cleaned, filtered, and formatted in a structured
manner by data engineers. This could involve collecting data, converting
data kinds, and handling missing values.
Data
Storage:
After processing, data must be kept in a format that makes it easy to recover
and analyze. To store data safely and easily, data engineers create and
manage databases or data warehouses.
Data
Integration:
A lot of businesses work with data coming from different systems and
sources. Data engineers combine these inconsistent data into a unified
structure, facilitating cross-functional analysis.
Data
Quality and Monitoring:
Data engineers ensure the quality and accuracy of the data by implementing
data validation and monitoring processes. This helps detect and address
issues as they arise.
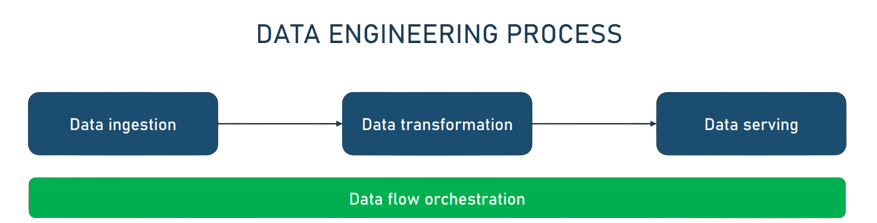
Difference between Data Engineers
and Data Scientists.
People usually misunderstand the
two terms. As we have discussed the concept of data engineering above. Data
engineers and scientists often work closely together but serve
different functions. While Data Engineers develop, test, and
maintain data pipelines and data architectures. Data Scientists tease out insights from massive amounts of
structured and unstructured data to shape or meet specific business needs and
goals.
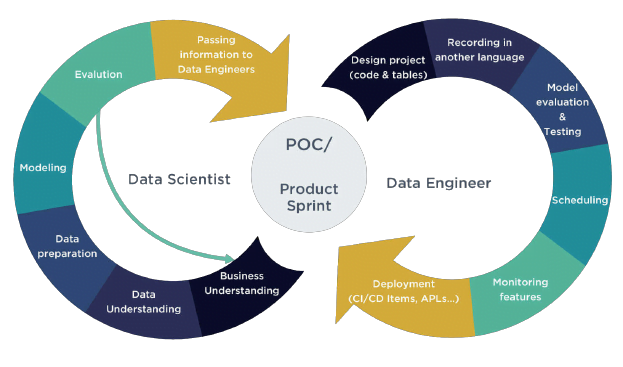
Why Is Data Engineering Important
in Every Field?
With Technological advancements, all the work has been shifted from manual work to digital or automated work. The volume of data has been increasing enormously for the past few decades. With this increase in the data, there is a need for such systems to fetch, store, and manipulate the essential data in every field. For this purpose, there is a never-ending need for Data Engineers in every field of life.
Informed Decision-Making: Data engineering is crucial for informed decision-making in any field. Structured and accessible data allows organizations to draw insights, identify trends, and make data-driven decisions that can lead to improved efficiency and competitive advantages.
Business Intelligence: Data engineering is fundamental to
building business intelligence systems. It helps in the development of
dashboards, reports, and analytics tools that offer a clear and comprehensive view
of an organization's performance and help in strategic planning.
Automation and Efficiency: Data engineering plays a pivotal
role in automating data-related tasks. By establishing reliable data pipelines
and transformation processes, organizations can save time and resources,
reducing manual data entry and data processing efforts.
Personalization and Customer Experience: Many industries, including
e-commerce and digital marketing, use data engineering to personalize customer
experiences. Organizations can tailor their products, services, and marketing
strategies to individual preferences by collecting and analyzing customer data.
Compliance and Security: In fields like healthcare and
finance, data engineering is essential for maintaining data security and
compliance with regulatory standards. It ensures that sensitive information is
protected and accessed only by authorized personnel.
Innovation and Research: Data engineering is a driving
force behind innovation in fields like healthcare, scientific research, and
technology. It allows organizations to collect, analyze, and draw insights from
massive datasets, leading to breakthroughs and advancements.
Predictive Analytics: With the help of data engineering, predictive analytics models can be developed. These models can forecast future trends, customer behavior, and market dynamics, aiding businesses in adapting to changing conditions.
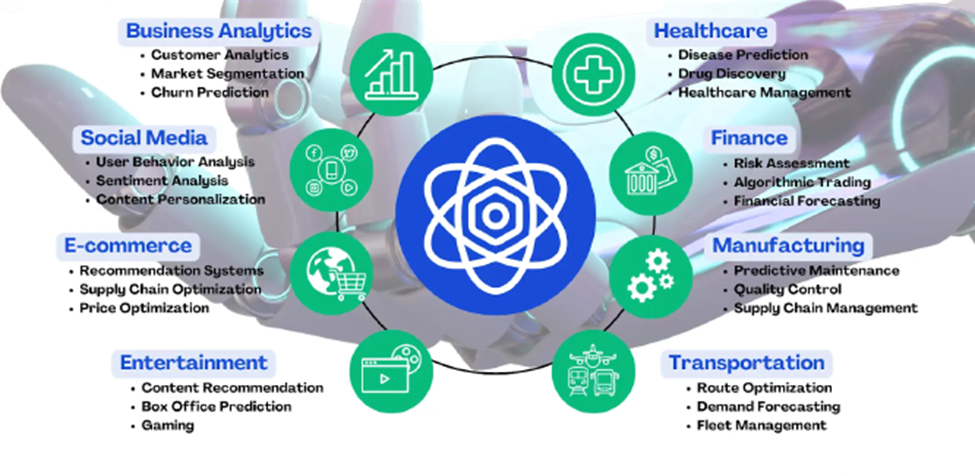
Summary:
This article emphasizes the critical
role of data engineering in the modern data-driven landscape. It highlights the
exponential growth of data from diverse sources and its potential to drive
decision-making, innovation, and business success. In its raw form, data is
often chaotic, underscoring the need for data engineering. The article delves
into the core responsibilities of data engineers, including data extraction,
preparation, pipeline design, and infrastructure management.
Data engineering is defined as the discipline that transforms raw data into a structured, manageable, and actionable format. It emphasizes the symbiotic relationship between data engineers and data scientists, with a focus on ensuring data quality and accuracy. The importance of data engineering in various fields is explored, highlighting its role in informed decision-making, business intelligence, automation, personalization, compliance, innovation, and predictive analytics. The article concludes by underscoring the ever-increasing demand for data engineers in our digital era
Dot Labs is an IT outsourcing firm that offers a range of services, including software development, quality assurance, and data analytics. With a team of skilled professionals, Dot Labs offers nearshoring services to companies in North America, providing cost savings while ensuring effective communication and collaboration. Visit our website: www.dotlabs.ai, for more information on how Dot Labs can help your business with its IT outsourcing needs. For more informative Blogs on the latest technologies and trends click here
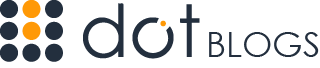