With the advancement of technology, the volume of data
also increased. In the early 2010s, with the rise of the internet, these
numerous increases in the data volume, velocity, and variety have led to the
term big data to describe
the data itself, and data-driven tech companies like Facebook and Airbnb
started using the phrase data engineer for the people performing these tasks.
Data has been part of our identity as humans since the
time of the ancient Romans. We’ve gone from 0% of the world on the internet to
59.5% of the world. 4.32 billion people with cell phones generate quite a huge
data feed. How has humanity dealt with the need to analyze this extraordinary
amount of data? We’ve come up with punch cards, relational databases, the
cloud, Hadoop, distributed computing, and even real-time stream processing to
try and manage this data.
In
recent years data silos have come out as a big issue for companies. Every big
organization consumes a large amount of data to perform decision-making. It
becomes possible with the assistance of a data engineer. Data engineers play a
crucial role in examining the infrastructure and performing relatable actions
on it.
Current
Trends of Data Engineering:
Data engineering is a constantly evolving field with new technologies and practices emerging faster than ever before. In recent years, several trends have appeared in the world of data engineering that are shaping the way data is stored, processed, and analyzed. Let’s explore some of the top trends in data engineering: Data Lake-houses, Open Table Formats, Data Mesh, DataOps, and Generative Artificial Intelligence.
·
Data
Lake-houses
Data Lakehouses represent a novel paradigm in the
realm of data storage and processing, integrating the most advantageous
attributes inherent in both data lakes and data warehouses. A Data Lakehouse
essentially fuses the high-performance, comprehensive functionality, and
stringent governance associated with data warehouses, with the scalability and
cost-efficiency advantages offered by data lakes. This transformative
architecture empowers data engines to directly access and manipulate data
residing in data lake storage, avoiding the need for costly, specialized
systems or the intermediary use of ETL pipelines for data replication.
The ascendance of the Data Lakehouse architecture is
primarily attributed to its growing popularity as it furnishes organizations
with a unified, singular vantage point encompassing all enterprise data. This
holistic view is readily accessible and amenable to real-time analysis, thereby
affording organizations a heightened capacity to extract valuable insights from
their data reservoirs, thereby enabling them to gain a distinctive competitive
edge in their respective domains.
·
Open
table formats
Open
Table Formats represent a pioneering standard in data storage and processing
that champions the cause of seamless interoperability across diverse tools and
platforms. In the past, each tool or platform adhered to its proprietary data
format, thereby posing formidable challenges when it came to the transfer of
data between systems or the analysis of data across different platforms. This
led to vendor lock-in and the creation of data silos.
Notably,
Open Table Formats such as Apache Iceberg, Delta Lake, and Hudi introduce a
table format that is precisely engineered for optimal performance and
encompasses a broad spectrum of data types. This pivotal development streamlines
the task of working with data from various sources and facilitates the
utilization of assorted tools for data processing and analysis.
Open
table formats enable data lakes to be as approachable as databases, by
providing a framework for interaction using an array of tools and programming
languages. A table format empowers the abstraction of disparate data files into
a unified dataset, effectively transforming data lakes into more structured and
manageable entities resembling tables.
·
Data
mesh
Data Mesh is a new approach to data architecture that
emphasizes the decentralization of data ownership and management. In a
traditional data architecture, data is centralized in a single repository and
managed by a central team. In a Data Mesh architecture, data is owned and
managed by individual teams or business units, and access to data is governed
by a set of shared standards and protocols.
Data Mesh enables organizations to
scale their data architecture by allowing different teams to manage their data and build their data products. This reduces the burden on the central
data team and enables faster data processing and analysis.
·
DataOps
DataOps and MLOps are methodologies
that bring together data engineering, data science, and machine learning. These
practices streamline the process of developing, deploying, and monitoring data
pipelines and machine learning models. By automating and orchestrating these workflows, organizations can
accelerate their data-driven initiatives and improve collaboration between data
engineers and data scientists.
The DataOps approach will enable organizations to implement automated data pipelines in their private, multi-cloud, or hybrid environments. The main objective of DataOps and MLOps is to accelerate the development and maintenance cycle of analytics and data models.
·
Generative
AI
Generative AI is a new field of AI that enables
machines to create content, such as text, images, and videos. This technology
has significant implications for data engineering, as it can be used to
generate semantics, dictionaries, and synthetic data, which can be used to
train ML models. This automation not only accelerates the data engineering
process but also reduces the risk of human error, thereby increasing overall
data quality and reliability.
Modern
data engineering is shifting towards decentralized and flexible approaches,
with the emergence of concepts like Data Mesh, which advocates for federated
data platforms partitioned across domains.
Future
Trends:
The future trend of the world is going to be
automated. In the next 5 years, the data is going to be fully automated and the
data is going to become the end product. the data gaps between the
organizations and users will be reduced. As a result, there will be an
increased use and demand for fully automated clouds, and hybrid
infrastructures, which will highly affect the future of data engineering.
It is predicted that the future of technology is
Artificial Intelligence. Such that all the applications nowadays are
self-generating and automated. In the field of data engineering, there is also
going to be a rise in the use of AI, AI-powered tools can analyze large
volumes of data, identify patterns, and generate optimized ETL workflows
automatically. This automation not only accelerates the data engineering
process but also reduces the risk of human error, thereby increasing overall
data quality and reliability.
That will help
in speeding up the data manipulation processes. The data engineers will be more
specialized and will give a variety of services to the organizations.
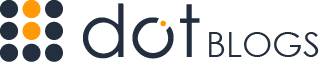
Dot Labs is an IT outsourcing firm that offers a range of services, including software development, quality assurance, and data analytics. With a team of skilled professionals, Dot Labs offers nearshoring services to companies in North America, providing cost savings while ensuring effective communication and collaboration.
Visit our website: www.dotlabs.ai, for more information on how Dot Labs can help your business with its IT outsourcing needs.
For more informative Blogs on the latest technologies and trends click here