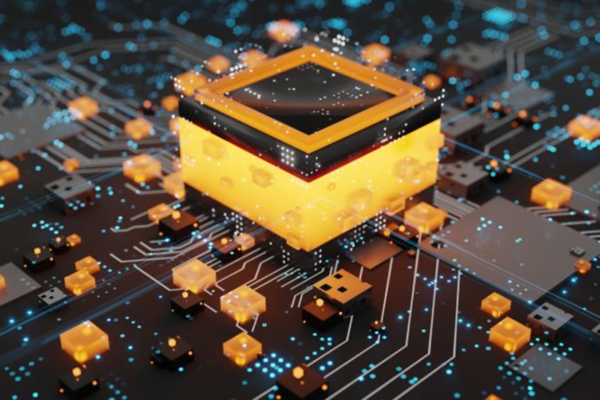
Beyond Bits and Qubits: Navigating the Landscape of Quantum Computing
In the dynamic realm of technology, “Beyond Bits and Qubits: Navigating the Landscape of Quantum Computing” explores the transformative potential of quantum mechanics. Moving beyond classical bits, quantum computing employs qubits, harnessing superposition and entanglement for unparalleled computational power. The article delves into quantum’s potential across diverse domains, from optimization and drug discovery to machine learning and cryptography. However, it also outlines critical challenges, including decoherence and hardware development. Highlighting companies like IBM and Google, the text explores quantum computers in action and introduces three types: gate-based, annealers, and topological. As quantum computing unfolds, preparation through education and hybrid approaches becomes paramount, marking a leap into a new era of computational possibility.